One of the biggest challenges we face at eDiscovery AI is relaying to people just how good AI is at document review. Let’s not mince words:
IT IS BETTER THAN ANY HUMAN OR MACHINE I HAVE EVER SEEN IN 10 YEARS OF WORKING IN EDISCOVERY.
If I’m telling you that this tool is so good you will never need contract reviewers again, how can I demonstrate that? Let’s show some examples!
For this one, we are going to dive into the Jeb Bush dataset and talk about Terri Schiavo (We are only here for the document review, and nothing written should be interpreted as any form of a political statement).
The first step is identifying our issues. We are looking for these 3 issues:
-
- Any documents that discuss the “Terri Schiavo situation.” I loosely describe it as a situation because I really want any documents that discuss her or the related legal battles or policy discussions around euthanasia.
- Any documents where a person argues that Terri’s husband should have a right to remove her feeding tube and allow her to die.
- Any documents where a person argues that Terri’s feeding tube should not be removed, and she should not be allowed to die.
Now we need to draft our instructions. After minimal thought, I went with the following:
"All documents that contain any discussion about Terri Schiavo or euthanasia"
"All documents that contain any argument that Terri Schiavo should be allowed to die"
"All documents that contain any argument that Terri Schiavo should NOT be allowed to die"
We run those instructions against the 1,000 documents we need to review and AWAYYYYYYYY WE GO!
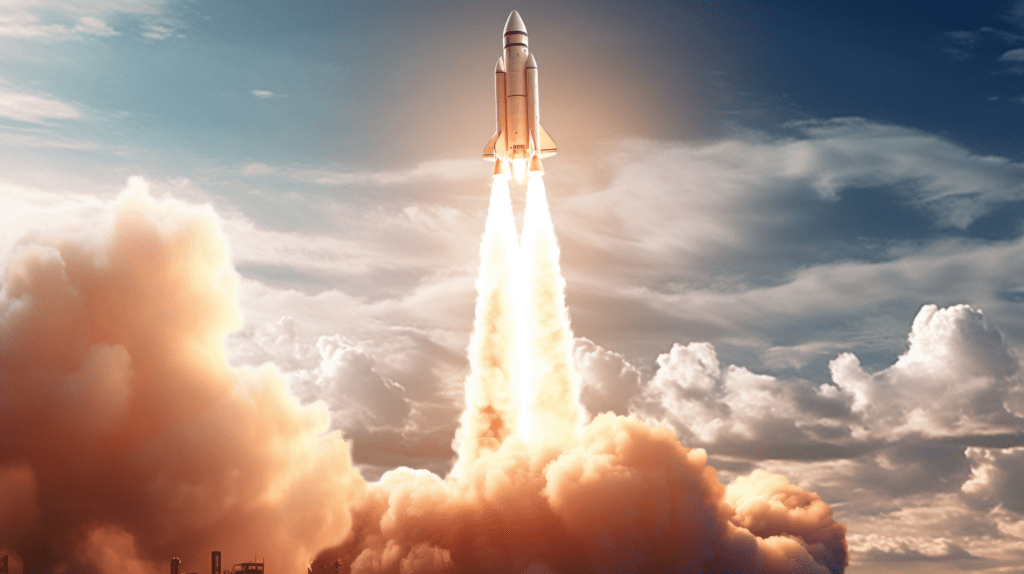
It doesn’t take long before we have our results. Let’s take a look…
Not a bad start. We knew that 500 docs were Relevant (based on our answer key), so things are looking pretty clean. 4 docs exceeded the default file size limit, and we don’t see any reason to change it right now so this is great.
Since these documents have already been coded, so we can immediately see the quality of the results. Let’s check the confusion matrix!
A quick guide on the confusion matrix:
True Positive = Instances where the AI classified the document as Relevant and the Subject Matter Expert also classified the document as Relevant.
True Negative = Instances where the AI classified the document as Not Relevant and the Subject Matter Expert also classified the document as Not Relevant.
False Positive = Instances where the AI classified the document as Relevant but the Subject Matter Expert classified the document as Not Relevant.
False Negative = Instances where the AI classified the document as Not Relevant but the Subject Matter Expert classified the document as Relevant.
So far, so good:
A quick calculation shows that we have 98.8% recall 99.8% precision. With ZERO up front work. That is incredible!
Let’s look at the one False Positive, because that will be quick:
You can view the document here: 143214.pdf
Luckily, we don’t have to guess why the AI marked it as Relevant, we can check the explanation:
It doesn’t have much, but there is a clear mention of “Terri Shaviano” in the document:
While we aren’t going to nit-pick the answer key, we aren’t going to lose any sleep over the fact that AI considered this Relevant.
Now on to the dreaded False Negatives:
You can view the 6 documents here: 203558.pdf, 214055.pdf, 217539.pdf, 215338.pdf, 217482.pdf, 202247.pdf
Let’s address one at a time.
This document is short and sweet:
It’s clear this document is relevant, as it mentions “going the extra 20 miles for Terri,” but there isn’t much substantive. Let’s keep this one in the back of our mind.
This one doesn’t have much content, but it has me scratching my head. I don’t see any way this could be considered relevant:
Let’s chalk this one up as an error with the answer key. Nothing we need to do, as AI classified this document correctly.
This one is a stretch…
That’s it. That’s the entire email. I’m not mad that we missed this one, but I think I can find a way to get it next time…
Another one with very little content that is really hard to justify being relevant:
Another one that won’t keep me up at night, but I’m seeing a trend. The date of the emails is the only way to be able to realize this COULD be talking about Terri…
Last one. Not much to it:
I need to throw the review flag on this one. There is just no way to connect this document to Terri. Or is there…
So after reviewing these docs, there are only 4 false negatives that cause any concern (keep in mind our recall is almost 99%!!!), but we can do better!
Let’s make some small changes to our instructions. Since the date is really the only indicator we have to determine some of these, let’s add the date:
"All documents that contain any discussion about Terri Schiavo's situation, which heated up around February and March of 2005. Any discussion about Terri's right to life or her husband's right to remove her feeding tube should be classified as relevant."
"All documents that contain any argument that Terri Schiavo should be allowed to die"
"All documents that contain any argument that Terri Schiavo should NOT be allowed to die"
NOW WE ARE COOKIN’ WITH PEANUT OIL!
By providing more complete instructions, we were able to identify documents that are only relevant because of context that is not present from within the document. That is mind blowing.
Keep in mind that we ONLY looked at the most difficult outliers. Let’s look at some documents it got right the first time:
217798: I stared at this document for a long time trying to figure out why it was relevant before giving up and looking at the explanation. Can you tell what makes it relevant?:
The explanation makes it clear. Some googling proved that all the listed parties are the counsel in Terri’s legal case.
15753: Not only was this document tagged as Relevant to the Terri Schiavo issue, but it was also tagged as Relevant to our 3rd issue:
214555: Another similar instance. This document was properly tagged as Relevant for the 1st and 3rd issue:
216486: This one was Relevant to the 1st and 2nd issue. And it provides a very clear explanation:
All in all, we were able to return 100% of the relevant documents with only a few minutes of work.
Show me another predictive coding solution that can do this.